The following first appeared in the Substack of Eric Topol, MD, called Ground Truths.
This week a new study using chest X-rays capped off a crop of many others for using medical scans for unintended diagnostic purposes, for which the term "opportunistic" is getting adopted.
The New Study
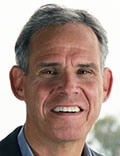
The atherosclerotic cardiovascular disease (ASCVD) risk score, based on 9 variables, is the most frequent way clinicians quantitatively gauge heart disease risk for patients over the next 10 years for the major adverse CV events (MACE) of heart attack, stroke and cardiovascular death. You can use the nomogram to quickly calculate your score right now. The main output is categorization of risk into 4 groups and recommendation about statin use. Details for which statin and dose are provided in the link.
Jakob Weiss and colleagues hypothesized that the ASCVD could be derived from the chest X-ray. That would have seemed highly improbable, a real reach, just a few years ago. They first developed a model using a very large cancer screening trial dataset with over 40,000 participants and >147,000 chest X-rays. With multi-year follow up for cardiovascular events from that large cohort, they went on to do independent testing of 2 different patient groups from the Mass General Brigham: one cohort of 2,132 patients with known ASCVD risk and another 8,869 with unknown risk (total of 11,001).
The striking bottom line result is that the AI of the chest X-ray for risk was better than the ASCVD! Better because it identified substantially more people who would benefit from statin therapy, the main output of the ASCVD risk score.
This has remarkable utility because the data to calculate the ASCVD is frequently missing (such as cholesterol values or systolic blood pressure). It was only available in 19% of the patients (2,132 of 11,001) in the current report. The chest X-ray is the most frequent type of medical image obtained — over 70 million in the United States per year alone! While we wouldn't order a chest X-ray to determine CV risk, think of the large number of people who would get this information "free" as a readout from their scan. Of course, this report needs to be independently replicated before it would be part of routine chest X-ray interpretations, but it gives you a sense of the rich information embedded in a scan that human eyes cannot detect, but somehow, inexplicably (for the most part, vide infra) at this point, digital, machine eyes can. And better primary prevention for heart disease over the next decade could be lifesaving for a substantial number of people who had this information.
Prior Studies
I've been stuck by many of them. Detecting diabetes from a chest X-ray was not something I would have anticipated. But deep learning (DL) from over 271,000 chest X-rays, > 160,000 patients, provided surprisingly high accuracy (AUC 0.84). To the credit of these researchers, there was a hunt for explainability, which turned out, by occlusion (analyze the data with and without regions) maps, to be related to fat pads in the chests.
Accurately determining the ejection fraction from the chest X-ray, as less than or greater than 40%, with an AUC of 0.92, via cross-training from echocardiography, along with many other cardiac parameters is another such achievement. As is estimation of the calcium score from the chest X-ray.
Imagine the chest X-ray of the future with readouts on heart disease risk, diabetes, and whether and what dose a stain medication should be considered.
There are more than 20 million chest CT scans done in the United States per year. But they aren't being used to detect pancreatic cancer or coronary artery disease risk or impute coronary artery calcium scores. Picking up heart disease risk from mammography via breast artery calcification is another example. And for abdominal CT scans, the opportunity afforded by AI to detect diabetes, cardiovascular risk, or pick up of pancreatic cancer far more sensitively.
What It All Means
Over the past 8 years, we've had ample evidence from hundreds of studies in radiology that deep learning AI can potentially be used to promote accuracy of medical image interpretation, across all different types of scans (X-rays, CT, MRI, PET, ultrasound). But that body of data is centered on a focused interpretation of the scan, such as pneumonia or a lung nodule on a chest X-ray. Opportunistic interoperation of medical scans presents something quite different.
This is a largely unanticipated windfall of AI applied to medical imaging — the ability to use machine eyes to uncover what human experts can't see, markedly enriching potential outputs of medical scans in the future. While that may provide much more bang for the buck, like a two-fer or three-fer of added findings outside the organ of interest, it's also possible it will lead to unwanted, incidental, false-positive findings that require further work-up. That's why it's vital to nail this down — to provide clear-cut benefit-risk assessment before trying to take advantage of it on a routine clinical basis. It would also be helpful to see more work as done in the chest X-ray detection of diabetes study that deconstructs and explains the model performance.
It's one more example of the power of machine eyes, which I've written about previously, like the retina being a window to multi-organ findings. It's in the continuum of unexpected AI outgrowths in medicine that should make us ponder more about what we are still missing that could be detected with the help of digital eyes. Or ears.
Thanks for reading and subscribing to Ground Truths. Please consider sharing this piece if you found it informative.